
Artificial intelligence sees more funding, but needs more people and better data
[ad_1]
The state of artificial intelligence is promising, and it is increasingly ready for real-life enterprises. But there are shortages of talent, lack of diversity in the field, and concerns about the handling the data that fuels ever-more-sophisticated algorithms.
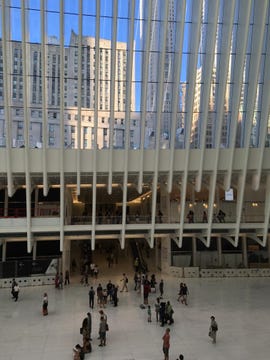
Photo: Joe McKendrick
These are some of the observations of Nathan Benaich and Ian Hogarth, prominent investors in artificial intelligence, who released their fourth annual and densely packed “State of AI” report reviewing developments in the field over the past year. While the report focuses on AI academia and specific advancements in medicine and other areas, there are important developments raised for those seeking to leverage AI and machine learning to move forward in building intelligent enterprises. “The under-resourced AI-alignment efforts from key organizations who are advancing the overall field of AI, as well as concerns about datasets used to train AI models and bias in model evaluation benchmarks, raises important questions about how best to chart the progress of AI systems with rapidly advancing capabilities,” Benaich and Hogarth state.
Some notable AI developments over the past year include the following:
- AI is now part of important real-life scenarios, including being applied to mission critical infrastructure such as national electric grids, automated supermarket warehousing optimization, drug discovery, and healthcare.
- “Transformers,” a neural network-based deep-learning architecture, have emerged as a general purpose architecture for machine learning, increasingly applied to natural language processing (NLP) and computer vision.
- Other developments mentioned include the rise of self-supervision in computer vision that require less training, and “textless” natural language processing based on Generative Spoken Language Modeling (GSLM), which enables the “task of learning speech representations directly from raw audio without any labels or text.”
- There has been record funding this year into AI startups, and IPOs for data infrastructure and cybersecurity companies that help enterprises retool for the AI-first era.
AI talent is a growing concern, as well as area of opportunity. “Computer research scientists, software developers, mathematicians, statisticians and data scientists saw an evolution of their employment that is far ahead of the general employed population,” Benaich and Hogarth state. “Computer science and engineering were the fastest growing undergraduate degrees over 2015 to 2018, accounting for 10.2% of all four-year degrees conferred in 2018. Their numbers increased by 34% and 25% respectively during the period, while the number of other awarded degrees increased 4.5% on average.”
Globally, Brazil and India are leading the way in AI employment growth, hiring more than three times more AI talent today than they were in 2017, matching or surpassing the hiring growth of Canada and the United States, they add.
The gender and racial diversity data within United States organizations radically differ between technical and non-technical teams, Benaich and Hogarth state. There is “a massive lack of gender diversity in technical teams, while a better balance is achieved in product and commercial teams. African Americans and Hispanics constitute a lower share of the AI workforce than their share in the general workforce, with the severest drop coming from technical teams. These teams also have the highest share of Asian workers.” Interestingly, on a global level, “almost 30% of scientific research papers from India include women authors compared to an average of 15% in the US and UK, and far greater than four percent in China.”
The venture capitalists point to concerns about managing big data in the AI space. “Careful data selection saves time and money by mitigating the pains of big data. Working with massive datasets is cumbersome and expensive. Carefully selecting examples mitigates the pain of big data by focusing resources on the most valuable examples, but classical methods often become intractable at-scale. Recent approaches address these computational costs, enabling data selection on modern datasets.”
Benaich and Hogarth point to the need for greater data quality data particularly in real-time situations, such as detecting or predicting life-threatening events. For example, they cite the threat of “data cascades,” defined by Google researchers as “compounding events causing negative, downstream effects from data issues.” These researchers warn “that current practices undervalue data quality and result in data cascades, pointing to factors such as “lack of recognition of the data work in AI, lack of adequate training, difficulty of access to specialized data for the studied region/population.” This calls for “developing metrics to assess goodness-of-data, better incentives for data excellence, better data education, better practices for early detection of data cascades, and better data access.”
The VCs also predict that the coming year may see the launch of a research company focused on artificial general intelligence (AGI), “formed with significant backing and a roadmap that’s focused on a sector vertical, which could potentially involve developer tools or a life science application.
[ad_2]
Source link